Faculty Profile
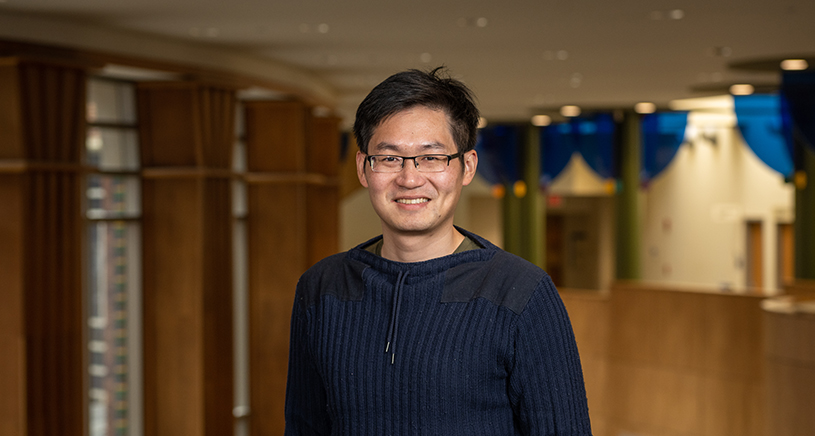
Zhenke Wu, PhD
- Associate Professor, Biostatistics
Dr. Wu's research is motivated by biomedical and public health problems and is centered on the design and application of statistical methods that inform health decisions made by individuals, or precision medicine. Towards this goal, he focuses on two lines of methodological research: a) structured Bayesian latent variable models for clustering and disease subtyping, and b) study design and causal methods for evaluating sequential interventions that tailor to individuals' changing circumstances such as in mobile health studies. He is committed to developing robust, scalable, and interpretable statistical methods to harness real-world, high-dimensional, dynamic data for individualized health. The methods and software developed so far have supported studies in diverse scientific fields including infectious disease epidemiology, autoimmune diseases, mental health, behavioral health, and cancer.
- PhD, Biostatistics, Johns Hopkins University, 2014
- BS, Mathematics, Fudan University, 2009
Research Interests:
Statistical: Hierarchical Bayesian models; Latent variable models; Nonparametric Bayes;
Bayesian scalable computation; Causal inference; Reinforcement learning.
Substantive: Precision medicine; Mobile health; Infectious diseases; Electronic health
records/claims data; Healthcare policy; Clinical trials.
Research Projects:
Bayesian Hierarchical Models for Using Mobile Technology to Individualize Care in
Mental Health
Structured Latent Variable Methods for High-Dimensional EHR and Administrative Data
Developing Innovative Statistical Framework to Integrate Multiple Verbal Autopsy Datasets to Estimate Cause-specific Mortality
Yao TH, Wu Z, Bharath K, Li J, and Baladandayuthapani V (2022). Probabilistic learning of treatment trees in cancer. Annals of Applied Statistics. Accepted.
Wang J, Fang Y, Frank E, Walton MA, Burmester M, Tewari A, Dempsey W, NeCamp T, Sen S, Wu Z (2022). Effectiveness of gamified competition as mHealth intervention for medical interns: a cluster micro-randomized trial. npj Digital Medicine. Accepted.
Li M, Park DE, Aziz M, Liu CM, Price LB, Wu Z (2021). Integrating sample similarity information into latent class analysis: a tree-structured shrinkage approach. Biometrics. In press. doi: 10.1111/biom.13580.
Wu Z and Chen I (2021). Probabilistic cause-of-disease assignment using case-control diagnostic tests: a hierarchical Bayesian approach. Statistics in Medicine 40(4): 823-841. PMID: 33159360. doi: 10.1002/sim.8804.
Wu Z, Casciola-Rosen L, Rosen A, Zeger SL (2020). A Bayesian approach to restricted latent class models for scientifically-structured clustering of multivariate binary outcomes. Biometrics 77(4): 1431-1444. PMID: 33031597. doi: 10.1111/biom.13388.
Wu Z, Deloria-Knoll M, and Zeger SL (2017). Nested partially-latent class models (npLCM) for dependent binary data; estimating disease etiology. Biostatistics 18(2): 200-213. PMID: 27549120. doi: 10.1093/bio-statistics/kxw037.
4626 SPH I
1415 Washington Heights
Ann Arbor, MI 48109
Email: zhenkewu@umich.edu
Office: 734-764-7067
For media inquiries: sph.media@umich.edu
Areas of Expertise: Aging, Biostatistics, Child Health, Clinical Trials, COVID-19, Health Informatics, Infectious Disease, Mental Health, Precision Health, Substance Use